Predicting Compressive Strength of M20 Concrete with Partial Replacement of Coarse Aggregates by Nueva Ecija Sourced Recycled Portland Cement Concrete Pavement using XGBoost Algorithm
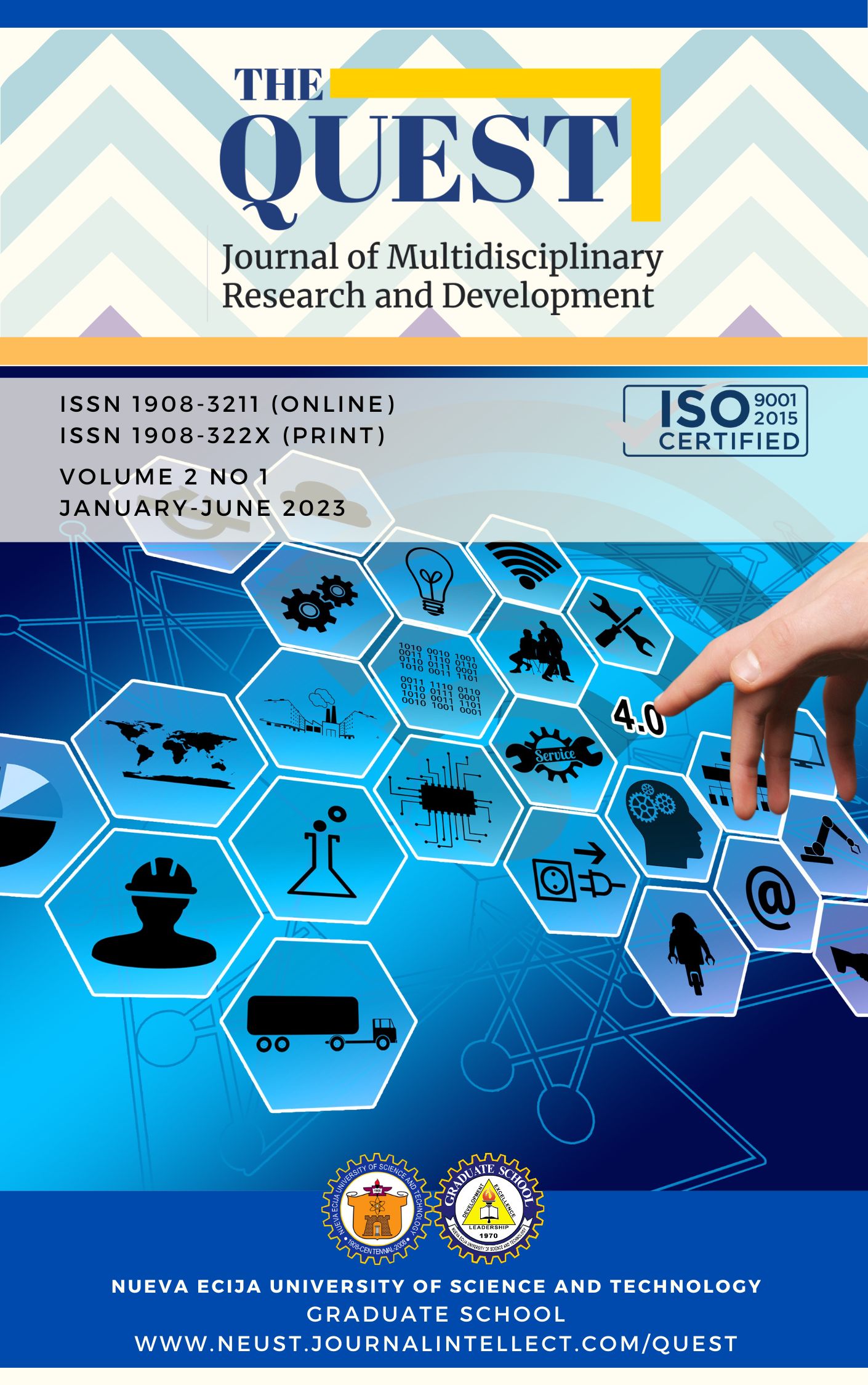
Published 06/30/2023
Keywords
- M20 Concrete,
- Materials,
- Recycled Concrete Aggregate,
- XGBoost,
- Machine learning
How to Cite
Copyright (c) 2023 The QUEST: Journal of Multidisciplinary Research and Development

This work is licensed under a Creative Commons Attribution-NonCommercial 4.0 International License.
Abstract
This research delves into the potential impact of replacing the coarse aggregates in M20 concrete with recycled concrete. The study aims to explore the possibility of recycling waste material from road construction to address sustainability challenges in the construction industry, especially in developing countries like the Philippines. The research adopts an experimental design involving preparing and testing recycled concrete aggregates, alongside sample preparation and testing. The study also employs the XGBoost machine learning algorithm to help predict the compressive strength of concrete. The XGBoost algorithm was trained using fine-tuned hyperparameters, and the model's evaluation was done using R-squared and Root Mean Squared Error. The research findings indicate a positive correlation between RCA content and compressive strength, with the average compressive strength of concrete mixtures increasing over time. The XGBoost outperforms the Multi Linear Regression model in predicting compressive strength, and incorporating RCA within the optimal range can enhance the strength properties of concrete. The study concludes that the XGBoost model with fine-tuned hyperparameters is reliable for predicting compressive strength, contributing to sustainable concrete production and reliable strength outcomes.
References
- Asian Development Bank (2017). SECTOR ASSESSMENT (SUMMARY): ROAD TRANSPORT – Road Improvement and Institutional Development Project (RRP PHI 41076). < https://www.adb.org/sites/default/files/linked-documents/41076-044-phi-ssa.pdf > accessed 23 December, 2022
- Azis, A. A. A., Memon, A. H., Rahman, I. A., Nagapan, S., & Latif, Q. B. A. I. (2012). Challenges faced by construction industry in accomplishing sustainability goals. 2012 IEEE Symposium on Business, Engineering and Industrial Applications. doi:10.1109/isbeia.2012.6422966
- Benachio, G. L. F., Freitas, M. do C. D., & Tavares, S. F. (2020). Circular economy in the construction industry: A systematic literature review. Journal of Cleaner Production, 121046. doi:10.1016/j.jclepro.2020.121046
- Esguerra, N.A., Amistad, F.T., & Rabena, A.R. (2008). Characterizing the Environmental Effects of the Quarrying Industry: The Case of Strategic Quarry Sites in the Ilocos Region. UNP Research Journal XVII (2008). < https://citeseerx.ist.psu.edu/document?repid=rep1&type=pdf&doi=e6a7da4774c91c3b5884453aa1ddfe725b941a59>
- Hawken, P., Lovins, E., and Levins, H. (1999) Natural Capitalism–Creating the Next Industrial Revolution, Little Brown and Co.
- Lee, J., Mahendra, S., and Alvarez, P. J. J. (2010). Nanomaterials in the Construction Industry: A Review of Their Applications and Environmental Health and Safety Considerations. ACS Nano 4(7), 3580-3590. DOI: 10.1021/nn100866w
- Mitchell, O. (2015). Experimental Research Design. In The Encyclopedia of Crime and Punishment, W.G. Jennings (Ed.). https://doi.org/10.1002/9781118519639.wbecpx113
- Mehta, K. (2001). Reducing the environmental impact of Concrete. Point of View. http://ecosmartconcrete.com/docs/trmehta01.pdf
- Montenegro, L.O. (2016). Environmental Trade-Offs from Coastal Reclamation: The Case of Cebu, Philippines. In: Olewiler, N., Francisco, H., Ferrer, A. (eds) Marine and Coastal Ecosystem Valuation, Institutions, and Policy in Southeast Asia. Springer, Singapore. https://doi.org/10.1007/978-981-10-0141-3_11
- Philippine Statistics Office (2022). Construction Materials Retail Price Index in the National Capital Region (2012=100): October 2022. < https://psa.gov.ph/price-indices/cmrpi/title/Construction%20Materials%20Retail%20Price%20Index%20in%20the%20National%20Capital%20Region%20%282012%3D100%29%3A%20October%202022>
- Simplilearn. (2023, 2 June). What is xgboost? An introduction to XGBoost algorithm in Machine Learning: Simplilearn. Simplilearn.com. https://www.simplilearn.com/what-is-xgboost-algorithm-in-machine-learning-article
- Yeheyis, M., Hewage, K., Alam, M.S. Eskicioglu, C., and Sadiq, R. (2013). An overview of construction and demolition waste management in Canada: a lifecycle analysis approach to sustainability. Clean Technology Environmental Policy 15, 81–91 (2013). https://doi.org/10.1007/s10098-012-0481-6